The Importance of Labeled Image Datasets in Today's Business Landscape
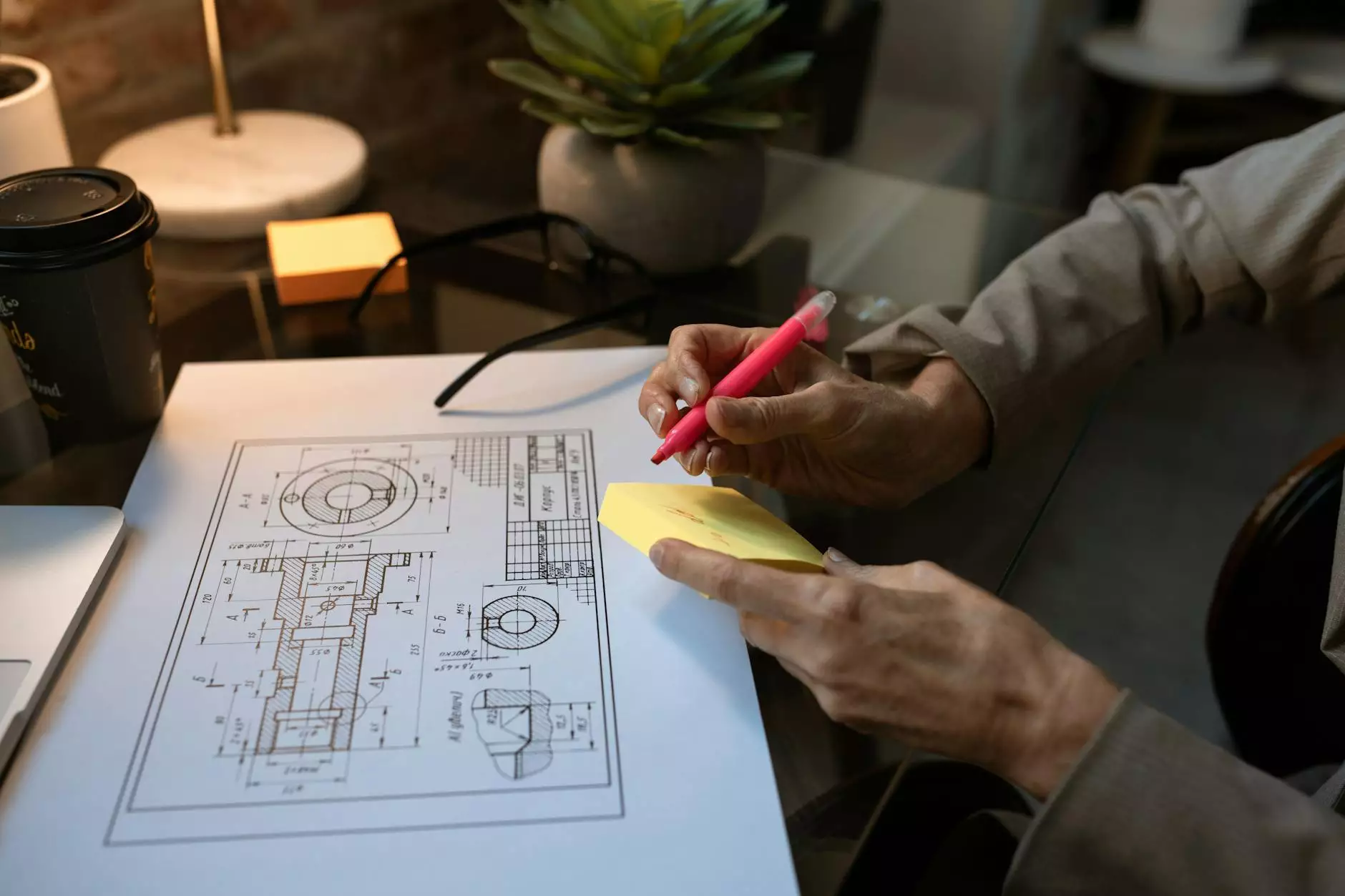
In the age of data-driven decision-making, labeled image datasets have emerged as a critical asset for businesses seeking to harness the power of artificial intelligence (AI) and machine learning (ML). As organizations increasingly turn to data to inform their strategies and operations, the demand for high-quality labeled data has never been higher. This article explores the significance of labeled image datasets, the tools and platforms available for data annotation, and the transformative impact of such datasets on business outcomes.
Understanding Labeled Image Datasets
The term labeled image datasets refers to collections of images that have been tagged or annotated with relevant information. This process is essential for training machine learning models, particularly in computer vision applications where algorithms need to learn from visual data. A labeled dataset typically includes:
- Images - The visual data itself.
- Annotations - Metadata providing context, such as object bounding boxes, segmentation masks, or textual descriptions.
- Categories - Defined labels that indicate whether an image contains specific objects or features.
Why Labeled Image Datasets Matter
Labeled image datasets are crucial for several reasons:
1. Enhanced Model Accuracy
High-quality labeled datasets serve as the foundation for training robust machine learning models. The more accurately labeled the images are, the better the model can learn to differentiate between various elements, leading to enhanced accuracy in predictions and classifications.
2. Speeding Up the Development Cycle
Access to comprehensive labeled image datasets significantly accelerates the model development cycle. With pre-annotated data, AI developers can move quickly from prototyping to deployment, ensuring faster time-to-market for their applications.
3. Reducing Costs
Investing in high-quality labeled datasets can reduce the long-term costs associated with model training and retraining. By utilizing existing datasets, companies can avoid the expenses linked to data gathering and annotation from scratch.
Applications of Labeled Image Datasets in Business
The applications of labeled image datasets span various industries, providing immense value:
1. Retail
In the retail sector, businesses use labeled image datasets to enhance customer experiences and streamline operations. For instance, computer vision algorithms can analyze product images for inventory management, and customer interaction can be improved through personalized recommendations based on visual cues.
2. Healthcare
In healthcare, labeled datasets are vital for training models that can assist in diagnostics. For example, medical imaging uses labeled datasets to identify tumors, lesions, and other abnormalities, facilitating more accurate and timely patient treatment.
3. Automotive
The automotive industry heavily relies on labeled image datasets for developing advanced driver-assistance systems (ADAS) and autonomous vehicles. Data collected from various environments and labeled accordingly allows these systems to recognize pedestrians, road signs, and obstacles.
The Role of Data Annotation Tools and Platforms
To effectively create labeled image datasets, businesses employ various data annotation tools and platforms. These tools facilitate the process of adding and managing annotations across large datasets. Here’s a closer look at their significance:
1. Streamlined Annotation Processes
Data annotation platforms like Keylabs.ai provide user-friendly interfaces for annotators, streamlining the labeling process. With features such as:
- Drag-and-drop annotation tools
- Automated labeling suggestions using AI
- Collaboration features for teams
These functionalities greatly enhance productivity and reduce the time taken to prepare datasets.
2. Quality Control Features
Maintaining high-quality standards in annotation is crucial for the effectiveness of the datasets. Many platforms offer integrated quality control mechanisms, ensuring that annotations are accurate and consistent. This includes:
- Review systems where multiple annotators can check each other's work
- Automated quality checks that flag inconsistencies
3. Scalability and Flexibility
A robust data annotation platform enables businesses to scale their annotation efforts based on evolving needs. Whether dealing with thousands or millions of images, businesses can adapt their strategies promptly without losing efficiency.
Choosing the Right Data Annotation Partner
Selecting the right data annotation partner is crucial for businesses looking to leverage labeled image datasets effectively. Here are some factors to consider:
1. Expertise and Experience
Look for platforms with proven expertise in your specific industry, as this can significantly influence the quality of annotations produced.
2. Technology Integration
Choose a solution that integrates smoothly with your existing systems. API access can facilitate easier data management and workflow automation.
3. Customization Options
Every business has unique requirements. A flexible annotation tool that allows for customized workflows and labeling schemes can enhance your data preparation process.
Best Practices for Managing Labeled Image Datasets
1. Regular Updates and Maintenance
As datasets age, their relevance can diminish. Regularly updating and expanding your labeled datasets ensures that your models remain effective and accurate.
2. Comprehensive Documentation
Maintain thorough documentation of your dataset characteristics, including the labeling criteria and the processes employed for annotation. This transparency improves replicability and understanding among team members.
3. Utilizing Active Learning
Incorporate active learning techniques to optimize your dataset continuously. This approach involves training the model iteratively and selecting the most informative samples for further annotation, thereby improving efficiency and model performance.
The Future of Labeled Image Datasets
The future of labeled image datasets looks bright, with advancements in AI paving the way for more innovative uses. Here are some emerging trends:
1. Automation and AI-Powered Annotation
As machine learning algorithms advance, the automation of the annotation process will become more prevalent, reducing reliance on human annotators and decreasing costs. AI can assist in generating initial labels, which can then be fine-tuned by human experts.
2. Enhanced Collaboration Across Platforms
Future platforms will likely offer more collaborative features, allowing teams to work together seamlessly, regardless of their location. Real-time updates and integrations will foster better teamwork.
3. Diversity of Data Sources
The incorporation of diverse data sources will enhance the robustness of datasets. As businesses embrace various types of image data, from aerial imagery to in-store surveillance footage, the potential applications will expand significantly.
Conclusion
Labeled image datasets are shaping the future of business. By investing in quality data annotation tools and platforms like Keylabs.ai, organizations can unlock unprecedented insights, drive innovation, and gain a competitive edge in their respective fields. Embracing the power of labeled image datasets is not just an option; it's a necessity for businesses aiming to thrive in the digital era.